How AI is being used in disaster recovery and management
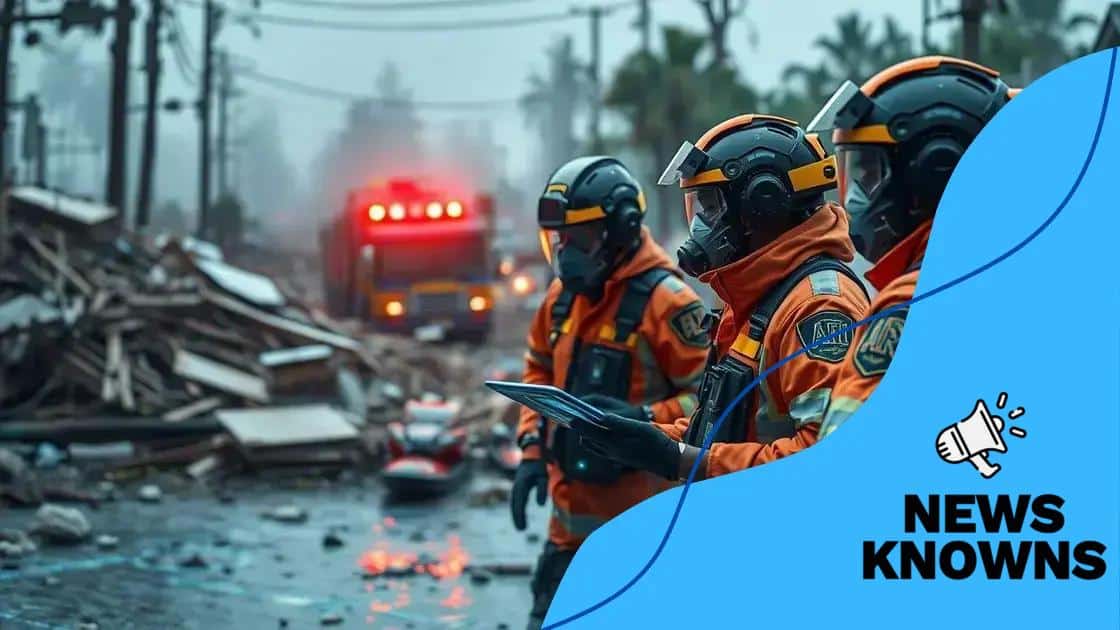
AI is being used in disaster recovery to analyze data in real-time, improve response times, predict risks, and enhance resource management, significantly boosting the effectiveness of emergency operations.
How AI is being used in disaster recovery and management is a vital topic as the world faces more frequent natural disasters. You might wonder how such technology can make a difference in critical situations. Let’s dive into the ways AI is transforming this field.
Understanding AI in disaster contexts
Understanding AI in disaster contexts is essential for improving emergency responses and resource management. As we face increasing natural disasters, it’s crucial to explore how technology can aid in these challenging situations. AI helps us predict, analyze, and respond more effectively to disasters, saving lives and resources.
The Role of AI in Disaster Prediction
One of the most significant contributions of AI is its ability to analyze vast amounts of data quickly. By using machine learning algorithms, we can identify patterns and predict when and where disasters might occur. This predictive power gives communities a chance to prepare and respond.
Key Applications of AI in Disaster Management
- Real-time data processing from various sources.
- Enhanced decision-making through predictive analytics.
- Improved communication during disasters.
- Resource allocation optimization based on data.
Moreover, AI tools can help streamline rescue operations by analyzing satellite images and social media data to locate victims. This technology not only enhances situational awareness but also supports teams in the field with actionable insights. With AI, we can track weather patterns more accurately, leading to timely evacuation alerts and better disaster preparedness.
In recent years, several governments and organizations have successfully integrated AI into their emergency response frameworks. For instance, AI-driven platforms have enabled rapid damage assessments after earthquakes or hurricanes, allowing for quicker restoration efforts. This technological integration makes it easier to respond efficiently to crises and allocate resources where they are needed most.
Ultimately, as we continue to enhance our understanding of AI in disaster contexts, we can develop better strategies to mitigate the effects of disasters. The future of disaster management will likely rely heavily on these technological advancements, ensuring that communities are safer and more prepared.
Real-time data analysis and decision making
Real-time data analysis and decision making are crucial components in managing disasters effectively. With AI technology at the forefront, agencies can now analyze incoming data streams almost instantly. This capability allows for timely decisions that can save lives and resources during critical situations.
The Importance of Real-time Data
In disaster scenarios, information changes rapidly. Having access to real-time data can mean the difference between a well-coordinated response and a chaotic situation. AI systems help process data from multiple sources such as satellites, drones, and social media feeds. This multidimensional approach ensures that responders have the latest information at their fingertips.
How AI Enhances Decision Making
- Data Integration: AI combines various data types to provide a complete picture of the situation.
- Predictive Models: By analyzing trends, AI can forecast potential developments in disasters.
- Resource Allocation: Optimal allocation of resources is facilitated through data insights, ensuring help reaches those in need swiftly.
- Communication: AI supports effective communication across different agencies, enhancing collaboration.
Moreover, AI models can simulate different disaster scenarios, allowing decision-makers to evaluate the most effective responses. By using historical data and current inputs, these simulations provide actionable insights. Decision-makers can evaluate various factors, like the best evacuation routes or resource deployment strategies, to ensure a well-formulated plan.
In addition to simulations, the capabilities of AI extend to alert systems, where real-time data analysis can trigger alerts for impending disasters. As soon as data suggests a threat, communities can receive warnings, allowing them to take necessary precautions. With timely alerts, individuals can often evacuate or prepare before disaster strikes.
Integrating real-time data analysis into disaster management enhances resilience in communities. Agencies that leverage these AI tools can react faster, adapt to changing circumstances, and ultimately improve the outcomes for those affected by disasters.
Predictive analytics for risk assessment
Predictive analytics for risk assessment plays a vital role in disaster management. By leveraging AI, organizations can forecast potential threats and assess risks before they become critical issues. This proactive approach allows for better planning and response strategies.
Understanding Predictive Analytics
Predictive analytics utilizes historical data and algorithms to predict future events. In the context of disasters, it involves analyzing data from past incidents to identify patterns. These insights help organizations understand what factors contribute to risks, enabling them to prepare effectively.
Key Benefits of Predictive Analytics
- Early Warning Systems: AI can alert authorities about potential disasters based on data trends.
- Resource Management: Helps allocate resources efficiently by predicting where they may be needed the most.
- Community Preparedness: Informs communities about possible risks, allowing them to develop safety plans.
- Improved Response Times: Faster decision-making based on accurate predictions can save lives.
Moreover, integrating predictive analytics with real-time data enhances overall effectiveness. For instance, during a storm, weather data can be analyzed to project its path and intensity. Emergency services can use this information to prepare evacuations and deploy resources where they will be most needed. This use of data not only ensures safety but also minimizes chaos during emergencies.
AI technologies can also assess vulnerabilities within communities, helping identify areas that may be at higher risk. This insight aids in designing targeted interventions, such as reinforcing infrastructure or conducting specific training programs for residents. By understanding potential risks, communities can foster resilience and ensure that they are better prepared to face disasters head-on.
Ultimately, the use of predictive analytics for risk assessment empowers organizations with knowledge and tools to respond effectively. By adopting these technologies, we enhance our capacity to manage disasters and protect lives.
Case studies: AI successes in disaster recovery
Exploring case studies of AI successes in disaster recovery shows how technology can profoundly impact communities in crisis. These real-life examples highlight the effectiveness of AI tools and strategies, proving their value in urgent situations.
AI in Hurricane Recovery Efforts
One notable success is seen in the aftermath of Hurricane Harvey in 2017. AI technologies were used to analyze satellite images and assess flood damage. This data helped disaster response teams efficiently allocate resources and assess which areas needed immediate assistance. The quick processing of information allowed responders to reach victims faster and provide necessary support.
Wildfire Management with AI
In California, AI played a critical role in managing wildfires. Using predictive modeling, AI systems analyzed weather patterns, vegetation data, and fire behavior to forecast fire spread. This information enabled authorities to issue timely evacuations, saving many lives. Firefighters could concentrate their efforts on the most at-risk areas, optimizing their resources.
Success Stories from Earthquake Relief
- Mexico City Earthquake (2017): AI algorithms analyzed social media posts to detect where people needed help after the earthquake hit.
- Japan Earthquake (2011): AI tools predicted aftershocks, helping emergency teams plan their response strategies effectively.
- Haiti Earthquake (2010): AI’s ability to process satellite images sped up the identification of damaged buildings for rescue operations.
- New Zealand Earthquake (2011): AI technologies assisted in evaluating infrastructure damage, leading to quicker restoration efforts.
These case studies demonstrate that AI successes in disaster recovery extend beyond mere predictions. They embody real-world impacts that improve response times and resource management. Through analytics and real-time data, communities can better prepare for and recover from catastrophic events.
Furthermore, as AI tools continue to evolve, their applications in disaster recovery are expected to grow. More sophisticated models will enhance predictive capabilities, ensuring that more lives are saved and communities are rebuilt more efficiently. By learning from these case studies, organizations can integrate AI effectively into their disaster recovery plans.
Challenges facing AI implementation
Challenges facing AI implementation in disaster recovery highlight the complexities that organizations must navigate. While AI technology holds great potential, various barriers can impede its effectiveness in critical situations.
Data Quality and Availability
One major challenge is the quality of data used for AI training and analysis. Accurate and relevant data is essential for predictive models to function correctly. However, in disaster scenarios, data may be incomplete or outdated. This can lead to faulty predictions and poor decision-making. Organizations need to focus on gathering high-quality data from reliable sources to ensure their AI systems perform optimally.
Integration with Existing Systems
Another significant hurdle is integrating AI tools with existing disaster management systems. Many organizations already use traditional methods, and adapting to advanced AI technologies can be difficult. Effective integration requires substantial investment in both time and resources. Moreover, staff may need extensive training to use new systems effectively. Proper training ensures that users can leverage AI capabilities to their fullest potential.
Ethical and Privacy Concerns
- Data Privacy: The collection of data raises concerns over how personal information is handled.
- Bias in Algorithms: If AI systems are trained on biased data, they can produce unfair outcomes.
- Accountability: Determining responsibility for AI failures can be complicated.
- Public Trust: Gaining public trust in AI interventions is crucial for their acceptance.
Additionally, ethical concerns surrounding AI use must be addressed. Issues like data privacy, biases in algorithms, and accountability for AI failures need careful consideration. Ensuring that AI systems are transparent and equitable will help foster public trust.
Furthermore, the rapid pace of technological change can be overwhelming for many organizations. Keeping up with new developments in AI requires continual learning and adaptation. Organizations must dedicate resources to stay informed about advances in AI technology and integrate best practices into their operations.
In conclusion, successfully overcoming the challenges facing AI implementation will require collaboration across sectors. By addressing data quality, ethical concerns, and integration issues, organizations can better leverage AI to enhance disaster recovery efforts.
FAQ – Frequently Asked Questions about AI in Disaster Recovery
How does AI improve disaster response times?
AI analyzes data quickly and provides real-time insights, which helps emergency teams react faster to changing situations.
What are the main challenges of implementing AI in disaster management?
The main challenges include data quality, integration with existing systems, and addressing ethical concerns related to privacy and bias.
Can AI predict natural disasters effectively?
Yes, AI uses historical data and advanced algorithms to predict patterns, helping to forecast possible disasters more accurately.
How can organizations ensure the ethical use of AI in emergencies?
Organizations can ensure ethical use by implementing transparent policies, addressing biases in algorithms, and prioritizing data privacy.